It is difficult to make predictions, especially about the future.” Nowhere is Yogi Berra’s deceptively brilliant aphorism more applicable than with financial planning. From households, to governments to financial institutions, allocating a budget for certain activities usually uses what’s happened in the past as a baseline with which to try to predict what will probably happen in the future. The trouble with this approach, especially for financial institutions, is that it makes no allowance for rapidly changing market conditions or for a full estimation of potential risks. Using multiple forecasting methods or frequent updates doesn’t help either, because those approaches still rely on historical data and the viewpoints of modellers, which tend to be skewed by their experiences. The solution? In my latest Global Association of Risk Professionals (GARP) column I explain how exhaustive reverse scenario analysis, driven by machine-learning, can help executives in charge of budget allocations make the right call for the year ahead.
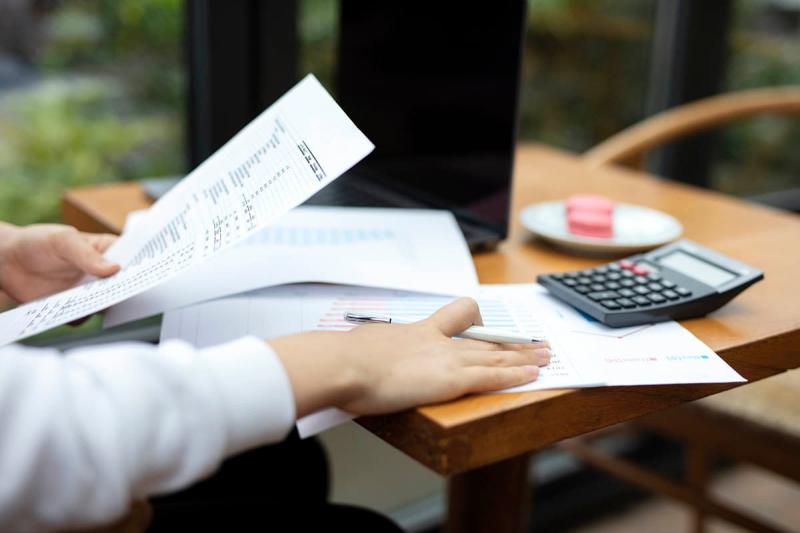